News
PFN Achieves Six-Fold Increase in Computational Speed for Deep Learning Workload with New Compiler for MN-Core Processor
Specialized compiler enables MN-Core to execute PyTorch workloads
2021.06.14
Correction (June 23, 2021):
“PFN’s tests showed that the computations using MN-Core and the new compiler were more than six times faster forinstance segmentationimage recognition” The workload tested was image recognition including object detection as well as instance segmentation. The graph labeling was also corrected accordingly.
TOKYO – June 14, 2021 – Preferred Networks, Inc. (PFN) has significantly increased the computational speed for practical deep learning workloads using its new specialized compiler for MN-Core™, a deep learning processor co-developed by PFN and Kobe University. The maximum computational speed was more than six times higher than PFN’s previous system that does not use MN-Core.
PFN will present the highly efficient and scalable deep learning approach made possible by the new compiler at the 2021 Symposia on VLSI Technology and Circuits.
PyTorch workloads compiled using PFN’s new compiler can be executed efficiently on MN-Core without major changes. PFN’s tests showed that the computations using MN-Core and the new compiler were more than six times faster for image recognition and nearly three times faster for graph processing than MN-2, PFN’s previous generation of supercomputer equipped with general-purpose GPUs. PFN sees the new capability as a competitive advantage in its research and development as deep learning datasets and models grow increasingly large and complex.
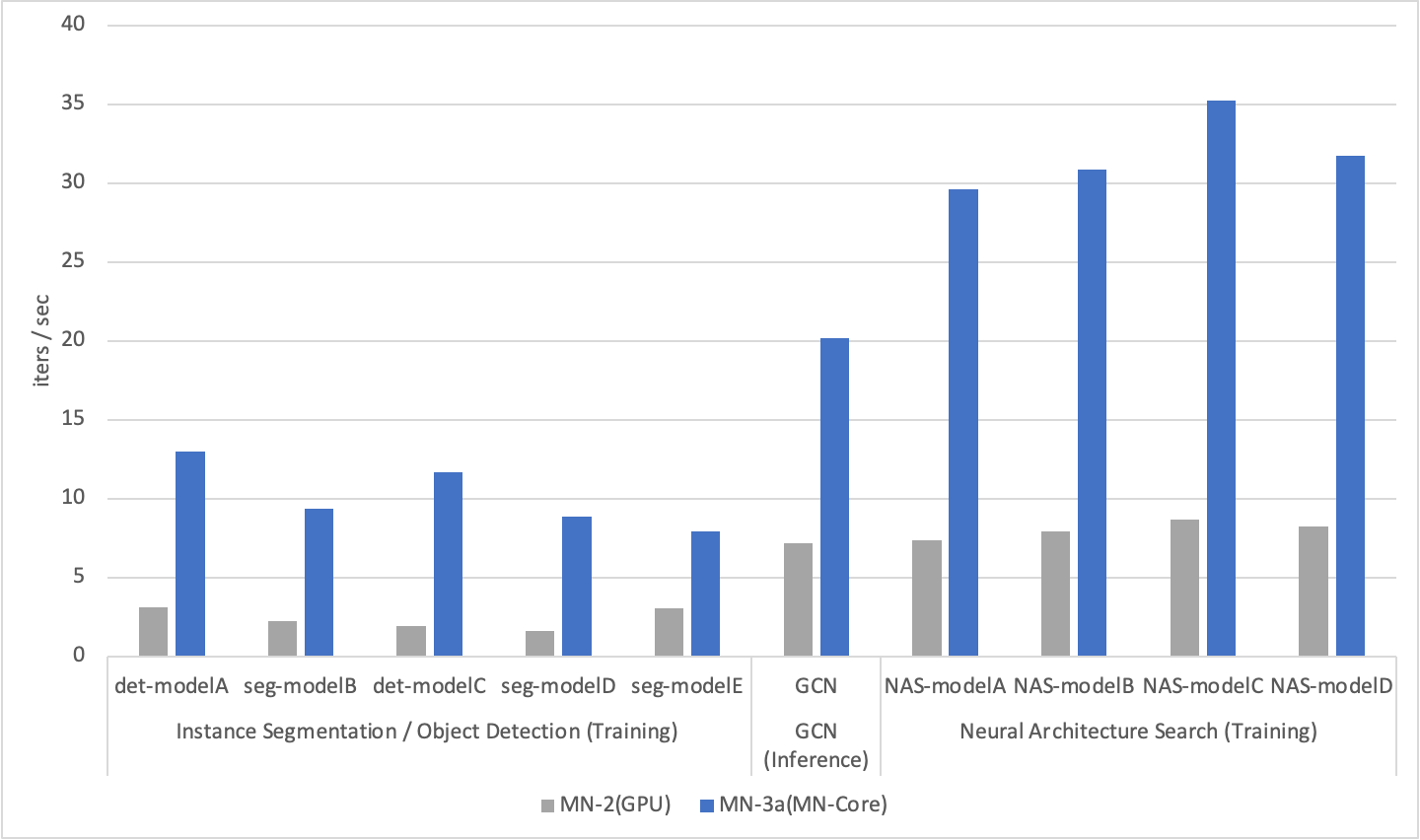
Comparison of performance per time unit of MN-2 and MN-Core-powered MN-3 for deep learning workloads
More technical details of the achievement are available on the PFN tech blog at:
https://tech.preferred.jp/en/blog/mncore-compiler-1/
MN-Core powers MN-3, PFN’s latest generation of supercomputer that started operation in May 2020 and topped the Green500 list of the world’s most energy-efficient supercomputers in June 2020. PFN has developed its software stack to maximize the potential of its hardware for deep learning, including MN-Core and MN-3.
Presentation at the 2021 Symposia on VLSI Technology and Circuits
Q&A session: 9:20-10:20 am Japan Standard Time on Tuesday, June 15, 2021
Session: CFS1 Energy-Efficient Machine Learning Processors
Presentation ID: CFS1-1
Title: MN-Core – A Highly Efficient and Scalable Approach to Deep Learning
Presenter: Ken Namura